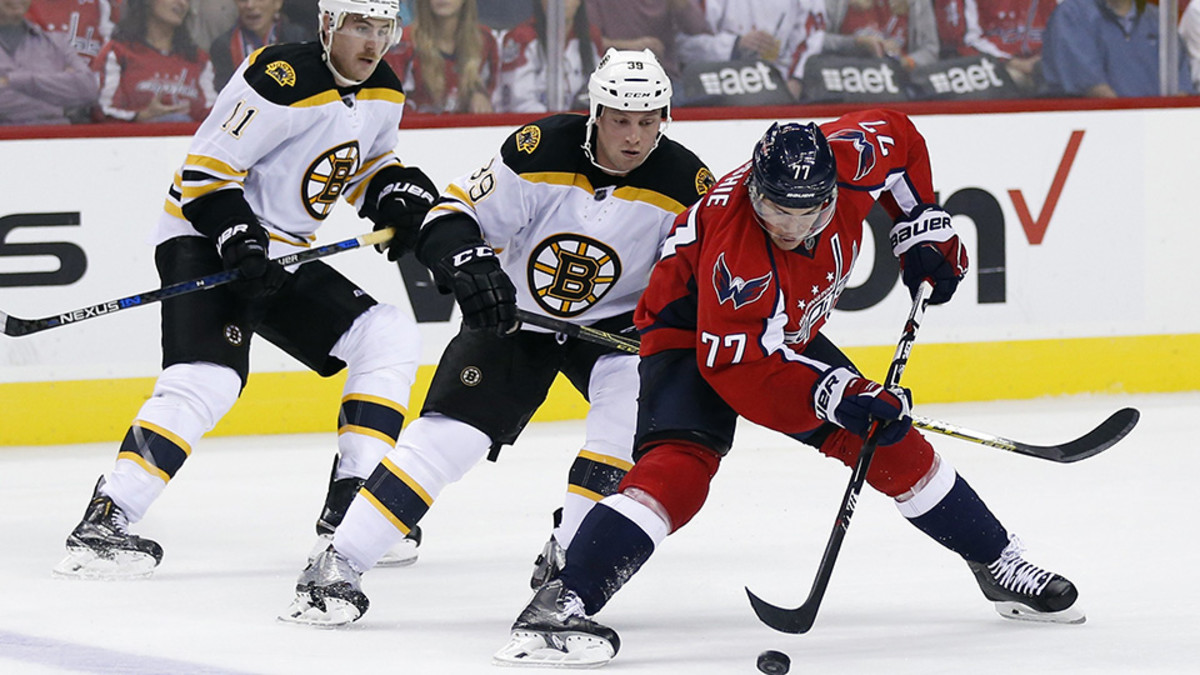
Can the first 10 games of a season predict a team's performance?
The NHL just passed the ten-game mark and analysts, fans, and teams alike are asking what their teams’ performances tell us about their playoff or Cup chances. But is it too early to have meaningful answers to those questions?
Think of it like this. At the start of the season, every goal, point, win, and loss is magnified. Three games in the Kings and Pens were 0–3 and the sky was falling. Would Sidney Crosby ever score again? Were the Kings a washed-up group whose heavy, north-south game had become a relic in the brand new age of speed, skill and fourth-liners that can do more than just punch other fourth liners in the face?
On the other end of the spectrum, the Sharks and Coyotes were 3–0. Would Martin Jones of the Sharks, who hadn’t allowed a goal in more than 178 minutes, win the Vezina? Would either of the Coyotes’ young guns, Max Domi and Anthony Duclair, carry the Coyotes to the playoffs?
Just how good are the 2015-16 Montreal Canadiens?
My how things change in just a couple weeks.
This just goes to show that (sample) size matters. What seems so important today will be a blip on the radar that nobody will even remember five months from now when the regular season ends.
Three games clearly tell us nothing. But do 10?
To answer that question we gathered more than seventy different stats going back ten years. These included both traditional stats like teams’ point totals, goal differentials, shooting and save percentages, as well as new-fangled advanced stats like scoring chance differentials, shot attempt differentials, zone starts, and penalties drawn and taken (stats courtesy of War-on-ice and puckon.net).
We then ran various regression analyses to determine which ones, ten games into the season, helped predict how teams would fare over the season’s final 72 games.
We started with team point totals. It turns out that even after ten games these don’t mean a heck of a lot. Sure, if you’re Columbus or Anaheim or Calgary you’re in trouble, but nobody needed us to tell them that. Beyond those outliers, though, team points after ten games predict only about 14.2% of the variation in teams’ point totals over the final 72 games. Bad news for teams like the Bruins, Senators, Coyotes, and Devils who are off to unexpectedly good starts, but may not finish the season as well as they’ve started it.
• ROUNDTABLE: Calder race reconsidered; how to boost scoring; more topics
Shooting and save percentages over ten games held almost no predictive power whatsoever. This shouldn’t be surprising since these stats can vary wildly over small samples.
It turns out that the most meaningful single stat after ten games is one that was just introduced this season called “EVSA,” which is an acronym of “Event, Venue, and Score Adjusted” shot attempt differential, and is based on the work of Micah Blake McCurdy. We’ll spare the gory details (if you’re curious you can find them at puckon.net), but EVSA takes regular shot attempt differentials and adjusts them based on the event (i.e., whether the shot missed, was blocked, was on goal, or resulted in a goal), the venue (i.e., home versus away), and score (teams trailing in score almost always attempt more shots than their opponents and vice versa).
What analytics predict for the 2015-16 NHL season
But even EVSA doesn’t tell us much after just 10 games, as it accounts for just 28.9% of the variation in teams’ point totals over the final 72 games.
The scatter plot graphs below illustrate the predictiveness—or lack thereof—of the ten-game point, shooting percentage, and EVSA stats. Each dot represents a team in one of the past 10 years. A stat with perfect predictiveness would show all the points along a straight line going up and to the right. For example, if ten-game point totals were perfectly predictive then teams with 15 points in the first ten games would always have more points in games 11 through 82 than teams with 14 points, and so forth.
That clearly isn’t the case.
The 10-game point graph shows the dots trending up and to the right, but hardly bunched tightly around an imaginary straight line. This depicts the 10-game point statistic’s weak 14.2% explanatory power.
The shooting percentage graph is pretty much an amorphous blob, which shows that ten game shooting percentage is unrelated to points over the next 72.
And the EVSA graph is more tightly bunched and shows a definite, albeit weak, trend toward the upper right. This depicts EVSA’s 28.9% explanatory power.
Go figure: stats to chew on as the NHL season nears the 10-game mark
But we can take the analysis one step further. As you might expect, looking at single statistics in isolation is often not as predictive as looking at a set of meaningful statistics considered together. So we mixed-and-matched various stats to see whether combinations were even more predictive than EVSA standing alone. We found that the set of statistics at the ten game mark that is most predictive of final point totals is EVSA together with points earned over the first ten. But even this combination predicts a measly 29.3% of the variation in teams’ point totals over the final 72 games.
In other words, a full 70% of the variation in teams’ performance over the final 72 games can’t be explained by teams’ performance over the first 10 games, at least using the more-than-seventy statistics we looked at. Apart from the teams at the very top and very bottom, ten games’ worth of stats tells us very little about how teams are going to finish the season.
But where’s the fun in that?
So we went ahead and predicted each team’s expected point totals over the final 72 games based on the formula produced by our points and EVSA combo analysis. The results are set out in the table, which is organized in order of projected points for games 11 through 82.
Team | Points After 10 Games | 10-Game EVSA | Predicted Points in Games 11-82 | Predicted End of Season Point Totals |
L.A | 14 | 57.9 | 93.24 | 107.24 |
MTL | 18 | 56.3 | 92.51 | 110.51 |
STL | 15 | 56.2 | 91.21 | 106.21 |
WSH | 16 | 55.6 | 90.74 | 106.74 |
FLA | 11 | 55.3 | 88.37 | 99.37 |
DAL | 16 | 53.1 | 87.17 | 103.17 |
NYR | 14 | 53.2 | 86.54 | 100.54 |
NSH | 16 | 52.5 | 86.31 | 102.31 |
T.B | 12 | 52.6 | 84.90 | 96.90 |
MIN | 15 | 51.3 | 84.21 | 99.21 |
CHI | 12 | 51.8 | 83.76 | 95.76 |
BOS | 13 | 51.4 | 83.58 | 96.58 |
WPG | 13 | 51.0 | 83.01 | 96.01 |
OTT | 12 | 50.7 | 82.19 | 94.19 |
NYI | 14 | 49.6 | 81.40 | 95.40 |
CAR | 8 | 51.2 | 81.35 | 89.35 |
VAN | 12 | 49.5 | 80.48 | 92.48 |
ARI | 11 | 49.7 | 80.37 | 91.37 |
S.J | 10 | 49.2 | 79.27 | 89.27 |
N.J | 11 | 48.4 | 78.52 | 89.52 |
TOR | 4 | 50.3 | 78.51 | 82.51 |
PHI | 10 | 48.0 | 77.56 | 87.56 |
PIT | 12 | 47.2 | 77.19 | 89.19 |
DET | 9 | 47.2 | 76.03 | 85.03 |
BUF | 6 | 46.4 | 73.72 | 79.72 |
ANA | 4 | 46.2 | 72.66 | 76.66 |
EDM | 6 | 45.4 | 72.29 | 78.29 |
CBJ | 4 | 45.2 | 71.23 | 75.23 |
CGY | 5 | 43.8 | 69.62 | 74.62 |
COL | 7 | 41.2 | 66.69 | 73.69 |
But take this table with a grain of salt.
First, to say that the predictions are rough would be a massive understatement. Remember, 70% of the variation in teams’ points over the final 72 games isn’t predicted by our model.
Second, the predictions are based solely on taking each team’s ESVA and 10-game point totals and plugging those into the formula derived from our regression analysis. The formula is based on a type of average taken across all teams, but it would be wrong to assume that in reality the formula fits each team equally well. Most importantly, the EVSA statistic doesn’t come anywhere close to fully accounting for teams’ abilities to convert shots into goals, especially after just ten games. So relying on EVSA, as our model does, leads to some predictions that common sense tells us are unlikely to pan out.
• NOTEBOOK: Team Canada’s safe choice; Marchand vexes Bruins; more
For example, the model tells us that Toronto will get more points over the last 72 games than nine other teams, including Pittsburgh, Anaheim, and Detroit. This is a function Toronto’s solid shot metrics. But the model doesn’t take into account that Toronto just isn’t as skilled as, well, just about any other team in the league. Which means that the Leafs won’t convert as many of their shots and, as a result, the model overstates Toronto’s predicted performance, probably by a lot. Same goes for Carolina. Maybe Florida as well, though not to the same extent.
The inverse is equally true. Even if Pittsburgh did continue with an EVSA of only 47.2 for the rest of the season, which we think is unlikely, Crosby, Malkin, Kessel, and company are going to do a lot more with its 47.2% than Toronto will do with its 50.3%. So the model understates Pittsburgh’s predicted performance.
But most importantly, the point for fans and for teams is that after ten games, nothing is decided. Analytics can identify red flags raised both by teams that are struggling and teams that have good records but who may nevertheless not be playing very well. The advantage of looking at the numbers this early is that there’s still time for teams to identify and correct problems that might not be seen using the eye test, or by looking at the standings alone.
The Department of Hockey Analytics employs advanced statistical methods and innovative approaches to better understand the game of hockey. Its three founders are Ian Cooper, a lawyer, former player agent and Wharton Business School graduate; Dr. Phil Curry, a professor of economics at the University of Waterloo; and IJay Palansky, a litigator at the law firm of Armstrong Teasdale, former high-stakes professional poker player, and Harvard Law School graduate. Visit us on line at www.depthockeyanalytics.com